How Does AI Reduce False Positives in AML?
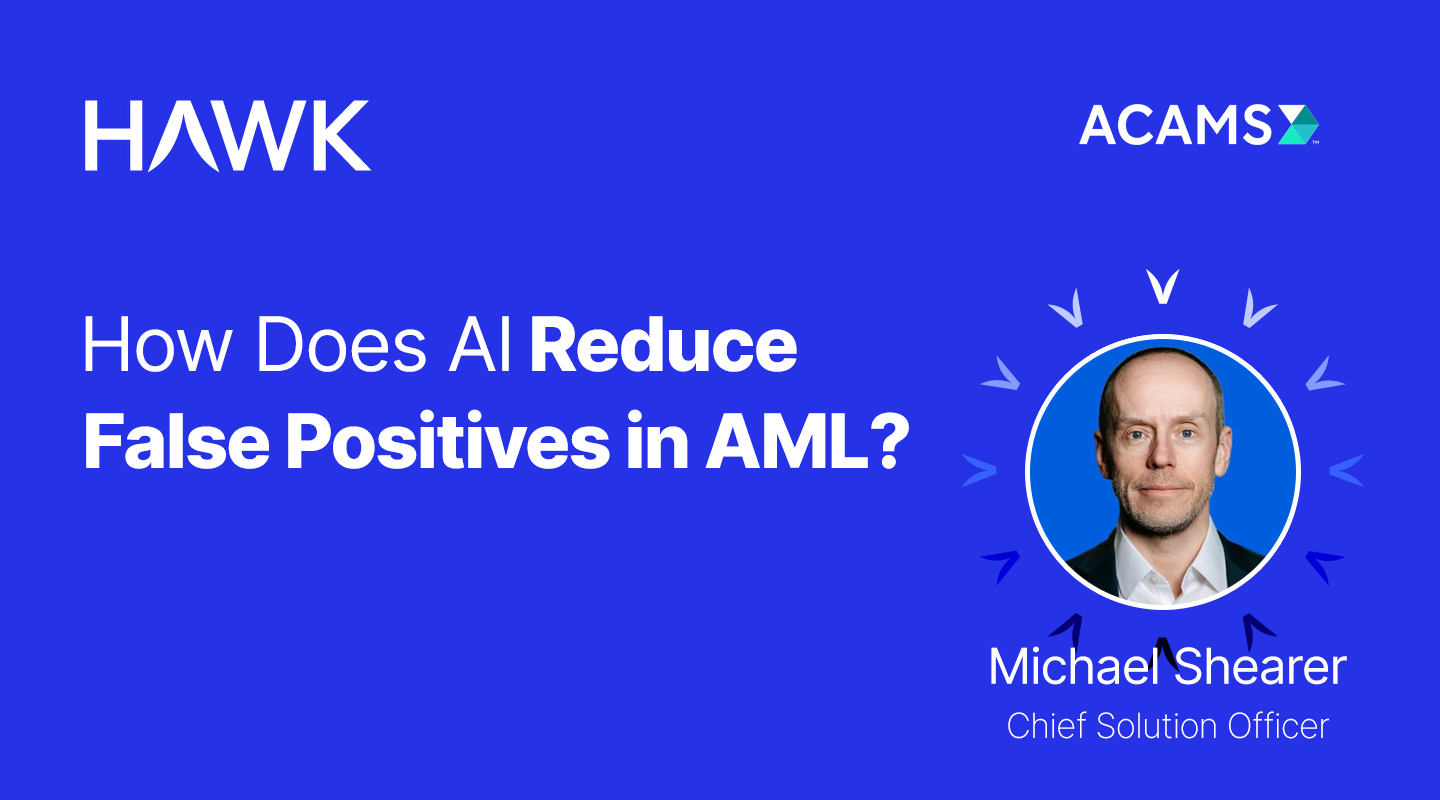
Artificial Intelligence (AI) is essential for detecting financial crime. But how does it improve anti-money laundering (AML) processes and operations? AI reduces false positives and finds more suspicious behavior than rules-based technology. By doing so, AI empowers financial institutions (FIs) to take a better-informed, risk-based approach to AML.
Hawk AI recently hosted a webinar with ACAMS where we talked about the practicalities of using AI for AML detection. We showed the benefits that AML professionals can expect to see from using AI and we discussed the challenges that are inherent to implementing AI for AML detection and how they can be overcome.
Watch the full webinar. (Access with free ACAMS registration.)
Key Topics
- Facing Rising Tides of False Positive AML Alerts
- Reducing False Positives with Contextual Information
- Mitigating AML Risk Earlier
The Expert Panel
- Moderator: Tobias Schweiger, Co-founder & CEO, Hawk AI
- Speaker: Michael Shearer, Chief Solutions Officer, Hawk AI & Former Group Head of Compliance Product Management, HSBC
- Facilitator: Sarah Runge, Executive Managing Director, K2 Integrity
Facing Rising Tides of False Positive Alerts
Many FIs that use rules-based AML technology suffer from an overwhelming volume of false positive alerts. These alerts are not truly suspicious, but AML investigators must examine them and clear them out of the case queue anyway. These alerts waste valuable investigator time and resources, hampering an FI’s efforts to achieve AML compliance and risk management goals.
In the example below, an FI uses rules-based technology to detect suspicious behavior. Out of one million customers, 1,000 are truly suspicious. The machine catches 99% of these suspicious customers. However, the system also mistakenly flags 9,990 good customers as suspicious, leading to a false positive ratio of 90.9%. It will take the FI an exorbitant amount of time and resources to investigate these cases; the FI could divert these efforts to investigate the 1,000 truly suspicious cases. These numbers are hypothetical, but the principle should ring true for FIs everywhere.
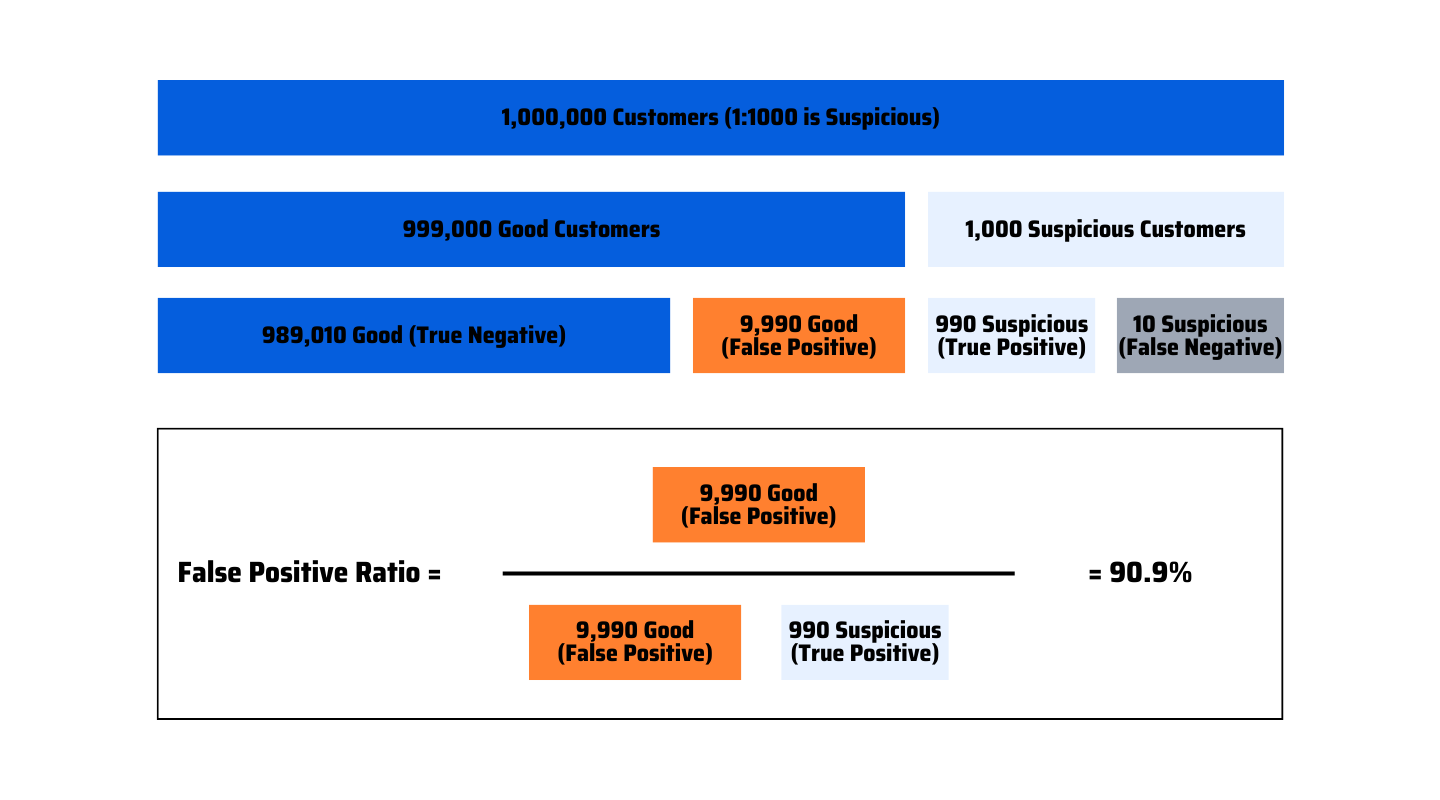
“What the industry has been struggling with for such a long time is that even if you build a really good mousetrap, a really good way of detecting financial crime, you still end up with this huge amount of false positives,” said Shearer.
Reducing False Positives with Contextual Information
AI applies more fine-grained rules than rules-based AML technology. We can think of AI as traditional rules “on steroids.” AI dynamically generates a network of interrelated rules for different segments of an FI’s customer portfolio. Because AI can apply more of these contextual filters simultaneously, it weeds out more false positive alerts.
The Rules-Based Approach
Consider three rules, each having a 10% false positive rate, deployed to detect suspicious behavior. When we apply these rules, the total false positive rate is 30%. The rate is high because all the rules act together, and they don't choose any specific type of behavior to look at. The same rule applies to every single customer.
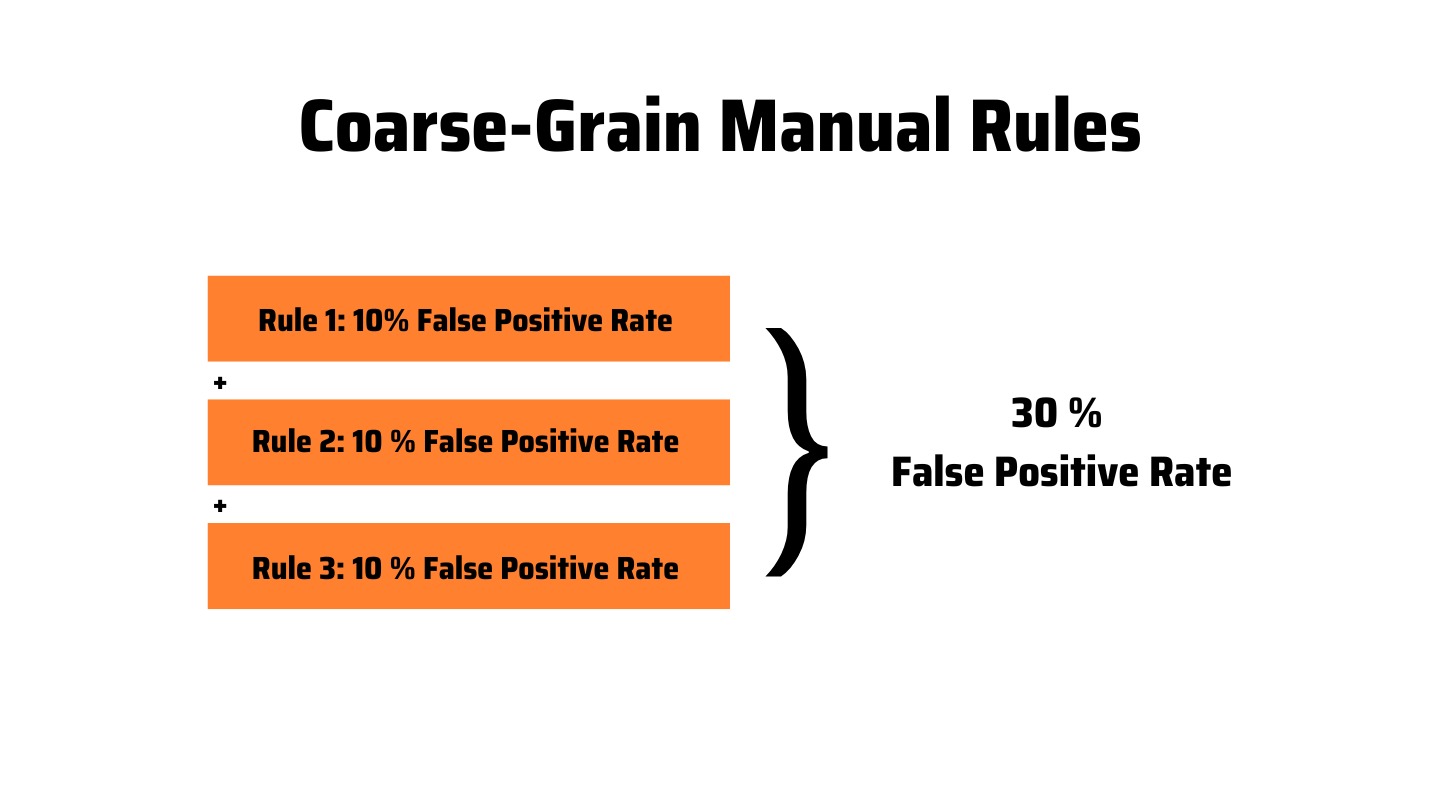
The AI Approach
AI generates a large set of fine-grain rules that look for specific combinations of behavior. AML investigators can tailor AI much more precisely to what a particular customer does. For example, with AI we can apply five rules, each with two conditions having a false positive rate of 10%. The rules only fire if both conditions are met, resulting in a false positive rate of 1% per rule. When we combine the five rules, we get an overall false positive rate of 5%. In this scenario, we’ve applied more rules and still reduced the false positive rate significantly. Imagine the resulting gains in efficiency and effectiveness when AI is applied at scale.
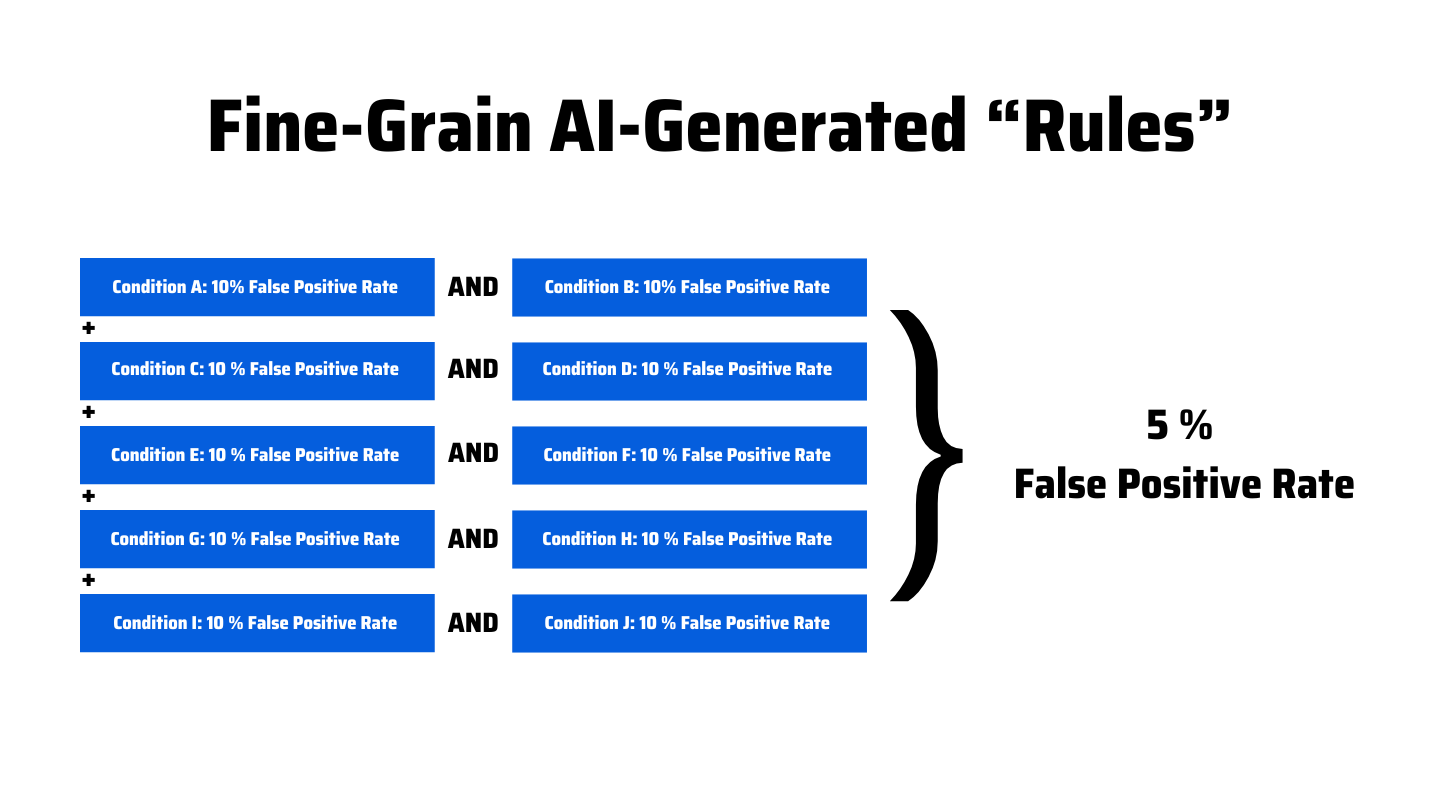
Mitigating AML Risk Earlier
Manual rule-tuning is difficult, and it takes time. The process looks something like this:
- Find a new behavior in individual casework.
- Collectively identify the new typology.
- Codify the typology into quantifiable behaviors.
- Set thresholds, segments, test, and tune.
- Deploy the rule to production.
AML risk accumulates throughout this time-consuming endeavor. On the other hand, the process to retrain an AI model is much faster:
- Find a new behavior in casework.
- Retrain model periodically.
- Deploy to production.
With AI, you don't have to go through the entire cycle manually. The machine automatically encodes emerging behavior and investigator expertise into a robust set of rules. This means you find AML risk earlier—and you can mitigate that risk earlier.
Hawk AI’s AML Technology
Hawk AI’s fine-grained AML technology supports FIs in the pursuit of compliance and risk management goals. Our AML technology helps FIs reduce false positive alerts, detecting more suspicious activity and using fewer resources. With our AML technology, FIs can mitigate AML risk sooner and intelligently filter and prioritize AML alerts.
To learn more, contact us today.