When Is It Appropriate to Use AI for AML?
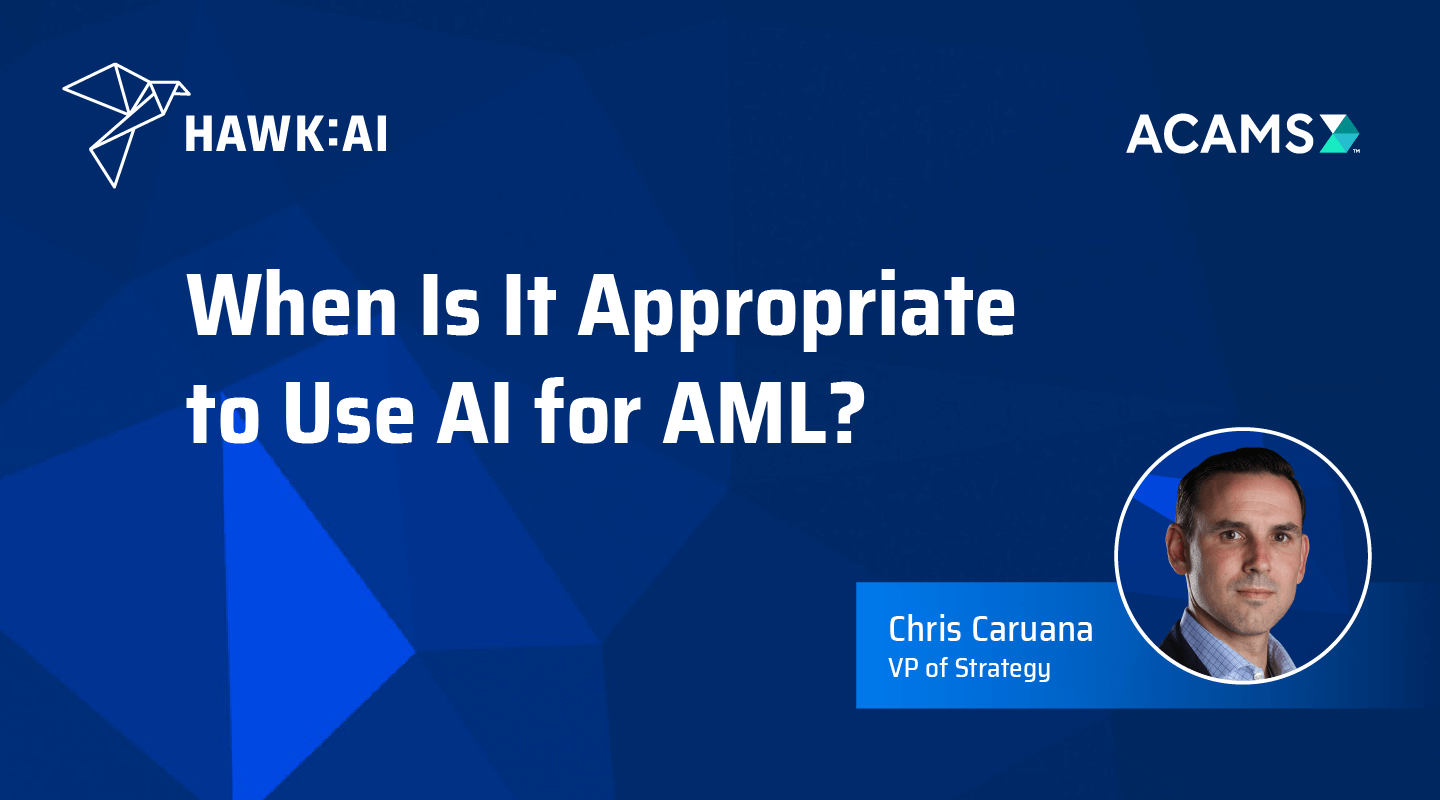
Artificial Intelligence (AI) delivers massive efficiency and effectiveness benefits to financial institutions (FIs) of all stripes. Even so, anti-money laundering (AML) managers and investigators have regulatory considerations to make. You may wonder: when is it appropriate to use this technology for AML purposes?
Many AML professionals are thinking about implementing AI and how they can measure its impact. On February 27, 2024, we discussed this topic with a panel of AML experts at an ACAMS New York Chapter event. The panelists spoke to an engaged audience and answered their questions about AI technology and its applications for AML.
The bottom line? AI technology is always appropriate for AML—when it’s designed to fit the purpose of detecting financial crime.

The Expert Panel
- Moderator: Nishi Gupta, Counsel, McDermott Will & Emery
- David Choi, Partner, Oliver Wyman
- Mehul Palan, Global Head of AML & Brand Risk Management Analytics & Detection, Paypal
- Yariv Ten-Ami, Global Co-Head of Financial Crime Compliance Transaction and Market Surveillance Strategy, Goldman Sachs
- Chris Caruana, VP Strategy, Hawk AI
In this article, we’ll discuss the following points:
- What AI Means in an AML Context
- What AI Use Cases Best Fit AML Purposes
- What the AI Development and Deployment Process Looks Like
- How Explainable AI Helps AML Professionals
- How to Measure AI’s Impact on AML Processes
What Does AI Mean in an AML Context?
AI refers to any model or algorithm that imitates human intelligence to accomplish specific tasks. In an AML context, AI technology enhances the detection of suspicious money laundering activity. AI delivers efficiency gains to AML teams via false positive reduction and alert prioritization, as well as effectiveness gains via anomaly detection and pattern recognition. AI is a tool that empowers AML teams to identify more suspicious behavior and focus investigative resources where they can make the greatest impact.
There are two primary categories of AI for AML professionals to consider:
- Machine Learning Models: Machine learning models can be either supervised or unsupervised. These models are trained on historic data and used to predict future outcomes. Machine learning models are typically used to characterize a portfolio and identify anomalies and outliers.
- Deep Learning Models (Including Large Language Models): Deep Learning and Large Language Models (LLMs) leverage multi-layer neural networks (NNs), often chaining multiple NNs together, to make predictions. LLMs learn patterns to interpret and generate text. These models require vast datasets and significant computational resources to train; they are often employed by institutions as pre-trained models (e.g., ChatGPT). FIs can leverage LLMs to accelerate and automate manual processes, improve the quality of documents and inputs, and gain insights into unstructured data (complaints, issues, breaches, risk assessment questionnaires, regulation library, and policies).
It’s critical for FIs to remember that AI does not replace a compliance professional’s judgement for decision-making. It enhances their capacity to make more quality decisions using fewer resources.
What AI Use Cases Best Fit AML Purposes?
AI technology has broad applications across multiple use cases. The following are the most valuable uses of AI for AML purposes:
- Policy/Program Setup & Maintenance: AML professionals can leverage AI to assess typically lengthy regulatory guidelines and legal obligations to inform an institution’s policies, procedures, and risk appetite.
- Transaction Monitoring: AML professionals can utilize the advanced capabilities of unsupervised and deep learning models to assess risk faster and more accurately, using a wide variety of data inputs.
- Due Diligence & AML Investigations: AML professionals can use the summarization capabilities of generative AI to improve consistency and accuracy of customer and case documentation, while introducing cost efficiencies.
- Regulatory & Compliance Reporting: AML professionals can employ AI to query regulations and prior reporting with models linked to vast data warehouses and external sources. This supports the drafting of policies and procedures in response to regulatory changes, and automates the analysis and interpretation of regulatory documents, legal texts, and public comments.
- Know Your Customer (KYC): AML professionals can apply AI to monitor the ongoing risk profile of their FI's customer base, enabling a perpetual state of KYC, rather than traditional periodic checks. AI can also interactively guide customers through the onboarding process, as well as summarize customer profiles based on data gathered during the process.
What Does the AI Development and Deployment Process Look Like?
Developing and employing AI technology for AML purposes involves an ongoing, iterative approach, which often looks like the following:
- Data Transfer and Cleansing
- Load data into the AI environment.
- Cleanse and prepare data for later stages.
- Rule and AI Simulation
- Segment portfolio.
- Tune and optimize rules.
- Set thresholds.
- Training of AI Models
- Detect criminal patterns.
- Detect false positives.
- Validation of AI Models
- Present AI model results.
- Approve model triggers.
- Deployment of AI Models
- Optimize performance.
- Push model live and monitor continuously.
- Ongoing AI Retraining
- Constantly collect operator feedback.
- Train model in automated pipeline.
This process ensures optimal accuracy and efficiency in the results of the AI model, demonstrating its fitness for AML purposes.
How Does Explainable AI Help AML Professionals?
AI models often operate as "black boxes," making it challenging for users and stakeholders (such as auditors, regulators, and compliance officers) to understand their underlying logic and alerting mechanisms. Regulatory expectations demand that compliance officers comprehend how alerts are generated by AI models and the rationale behind their decisions. This is why explainability is a crucial element to using AI for AML purposes. Explainability makes the logic behind AI models’ decisions transparent and understandable. Explainable AI delivers the rationale for its decisions in plain language, incorporating probabilities and contextual information. Users of Explainable AI technology can easily understand its outputs and defend them to regulators. Building explainability into AI increases trust in the soundness of the technology and its fitness for AML use. With additional regulation of AI technology on the way, explainability will be more essential than ever.
Providing context around alerts, including contributing features/risk factors and their importance for detection, is essential. AI methodologies should incorporate subject matter expert review and adaptation for greater accuracy and reliability. Continuous monitoring and governance are crucial to maintain model relevance and agility, allowing for corrective actions based on feedback and predefined key performance indicators (KPIs). Mapping AI models is more complex than it is for a traditional rule-based systems, as the AI models detect patterns using vast datasets and covering diverse AML risks. FIs must thoroughly document and analyze AI models against concrete detection outcomes to demonstrate their effectiveness in mitigating financial crime risks.
How Can You Measure AI’s Impact on AML Processes?
There are multiple ways to measure AI’s enhancement of AML operations, but the following are critical:
- Efficiency: Measure the reduction in time and human resources required for the investigation of AML cases. This can include metrics like processing time per transaction and number of false positives reduced.
- Accuracy: Evaluate how effectively AI algorithms identify suspicious activity compared to traditional rule-based systems. Metrics can include the number of true positives, false positives, false negatives, and true negatives identified.
AI and rule-based approaches are both fundamentally rooted in data science and statistics. However, AI detects more instances of financial crime and produces fewer false positive alerts. Using rules and AI together empowers FIs to better fine-tune their systems, balancing and maximizing both risk detection and alert efficiency.
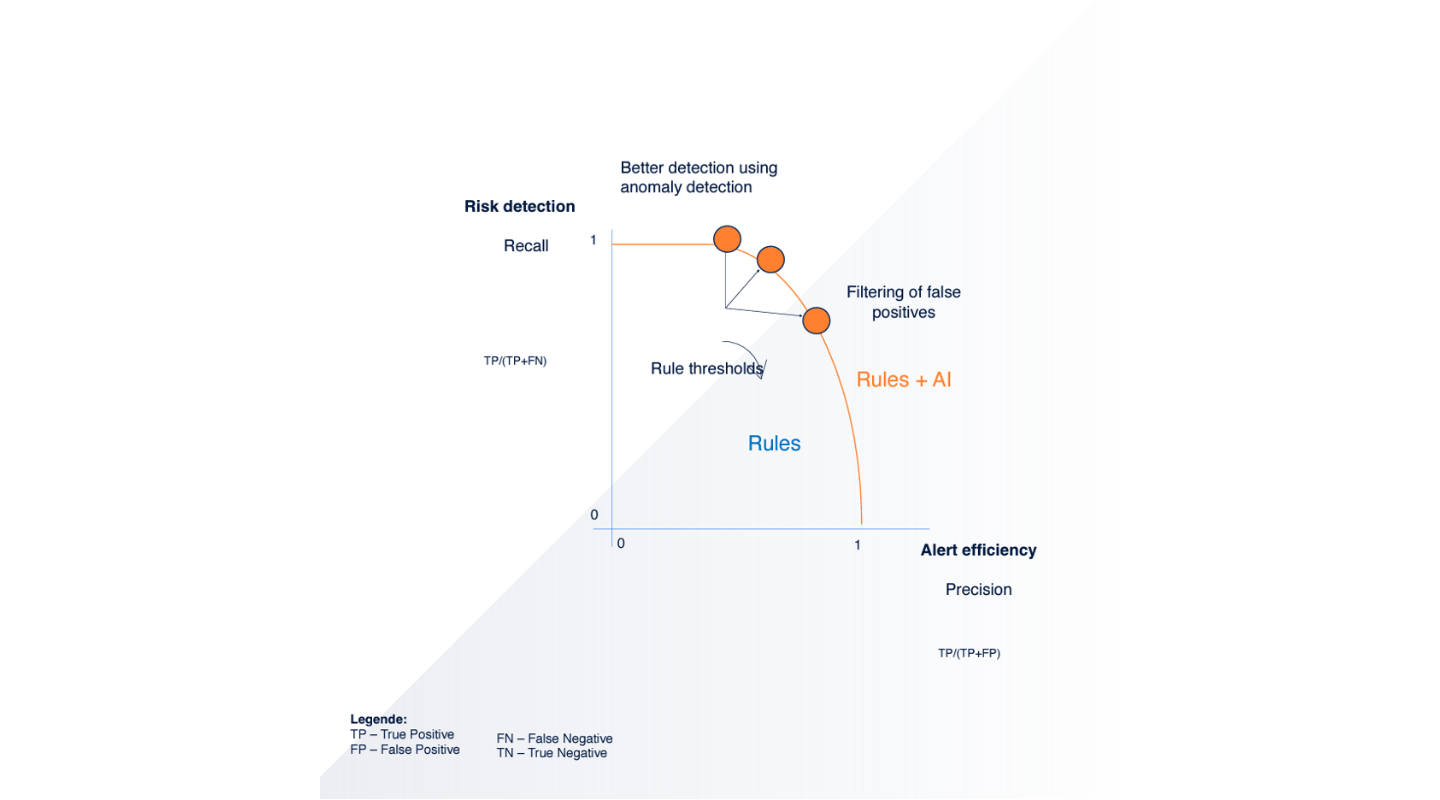
“Tracking KPIs like false positive reduction and true positive capture are good first steps,” said Caruana. “But you also must be looking at efficiencies around investigation times, SAR filing quality, and capacity against SLAs. Then you have to translate these improvements into the positive financial impact it's had on your company for internal consumption.”
Hawk AI’s AML Technology
Hawk AI’s AML technology empowers financial institutions to detect money laundering more effectively while using fewer resources, supporting the achievement of key AML compliance and risk management goals. Hawk AI’s technology is designed with data science and AML expertise to maximize its fitness for financial crime detection purposes. Our technology is fully explainable, providing natural language explanations and probabilities based on specific risk factors. Hawk AI’s technology is also highly configurable, allowing FIs to build and set rules and risk thresholds without a need for data science or coding expertise.
To learn more, contact us today.